09 - Saving, Loading Qiskit Machine Learning Models and Continuous Training
[1]:
# This code is at:
# https://qiskit-community.github.io/qiskit-machine-learning/tutorials/09_saving_and_loading_models.html
[2]:
import matplotlib.pyplot as plt
import numpy as np
from qiskit.circuit.library import RealAmplitudes
#from qiskit.primitives import StatevectorSampler as Sampler
from qiskit_machine_learning.optimizers import COBYLA
from qiskit_machine_learning.utils import algorithm_globals
from sklearn.model_selection import train_test_split
from sklearn.preprocessing import OneHotEncoder, MinMaxScaler
from qiskit_machine_learning.algorithms.classifiers import VQC
from IPython.display import clear_output
algorithm_globals.random_seed = 42
from quantumrings.toolkit.qiskit import QrSamplerV1 as Sampler
[3]:
sampler1 = Sampler()
sampler2 = Sampler()
[ ]:
[4]:
num_samples = 40
num_features = 2
features = 2 * algorithm_globals.random.random([num_samples, num_features]) - 1
labels = 1 * (np.sum(features, axis=1) >= 0) # in { 0, 1}
[5]:
features = MinMaxScaler().fit_transform(features)
features.shape
[5]:
(40, 2)
[6]:
features[0:5, :]
[6]:
array([[0.79067335, 0.44566143],
[0.88072937, 0.7126244 ],
[0.06741233, 1. ],
[0.7770372 , 0.80422817],
[0.10351936, 0.45754615]])
[7]:
labels = OneHotEncoder(sparse_output=False).fit_transform(labels.reshape(-1, 1))
labels.shape
[7]:
(40, 2)
[8]:
labels[0:5, :]
[8]:
array([[0., 1.],
[0., 1.],
[0., 1.],
[0., 1.],
[1., 0.]])
[9]:
train_features, test_features, train_labels, test_labels = train_test_split(
features, labels, train_size=30, random_state=algorithm_globals.random_seed
)
train_features.shape
[9]:
(30, 2)
[10]:
def plot_dataset():
plt.scatter(
train_features[np.where(train_labels[:, 0] == 0), 0],
train_features[np.where(train_labels[:, 0] == 0), 1],
marker="o",
color="b",
label="Label 0 train",
)
plt.scatter(
train_features[np.where(train_labels[:, 0] == 1), 0],
train_features[np.where(train_labels[:, 0] == 1), 1],
marker="o",
color="g",
label="Label 1 train",
)
plt.scatter(
test_features[np.where(test_labels[:, 0] == 0), 0],
test_features[np.where(test_labels[:, 0] == 0), 1],
marker="o",
facecolors="w",
edgecolors="b",
label="Label 0 test",
)
plt.scatter(
test_features[np.where(test_labels[:, 0] == 1), 0],
test_features[np.where(test_labels[:, 0] == 1), 1],
marker="o",
facecolors="w",
edgecolors="g",
label="Label 1 test",
)
plt.legend(bbox_to_anchor=(1.05, 1), loc="upper left", borderaxespad=0.0)
plt.plot([1, 0], [0, 1], "--", color="black")
plot_dataset()
plt.show()
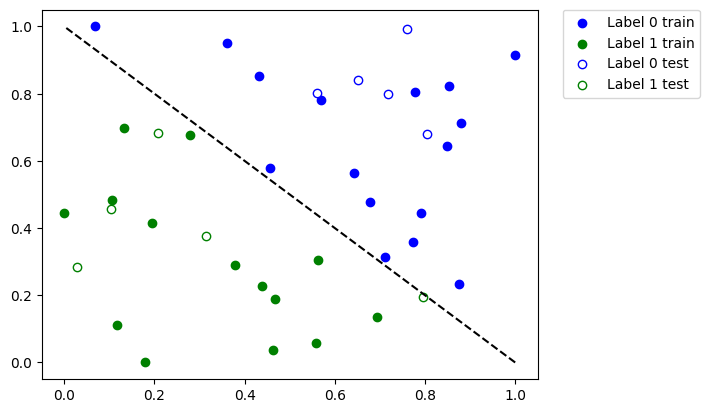
[11]:
maxiter = 20
[12]:
objective_values = []
[13]:
# callback function that draws a live plot when the .fit() method is called
def callback_graph(_, objective_value):
clear_output(wait=True)
objective_values.append(objective_value)
plt.title("Objective function value against iteration")
plt.xlabel("Iteration")
plt.ylabel("Objective function value")
stage1_len = np.min((len(objective_values), maxiter))
stage1_x = np.linspace(1, stage1_len, stage1_len)
stage1_y = objective_values[:stage1_len]
stage2_len = np.max((0, len(objective_values) - maxiter))
stage2_x = np.linspace(maxiter, maxiter + stage2_len - 1, stage2_len)
stage2_y = objective_values[maxiter : maxiter + stage2_len]
plt.plot(stage1_x, stage1_y, color="orange")
plt.plot(stage2_x, stage2_y, color="purple")
plt.show()
plt.rcParams["figure.figsize"] = (12, 6)
[14]:
original_optimizer = COBYLA(maxiter=maxiter)
ansatz = RealAmplitudes(num_features)
initial_point = np.asarray([0.5] * ansatz.num_parameters)
[15]:
original_classifier = VQC(
ansatz=ansatz, optimizer=original_optimizer, callback=callback_graph, sampler=sampler1
)
[16]:
original_classifier.fit(train_features, train_labels)
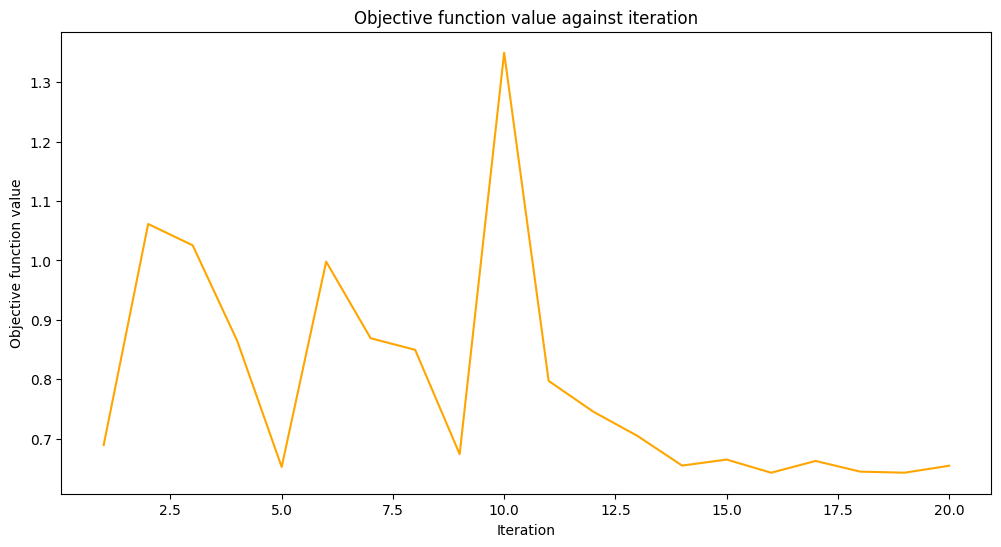
[16]:
<qiskit_machine_learning.algorithms.classifiers.vqc.VQC at 0x1ac7e885b10>
[17]:
print("Train score", original_classifier.score(train_features, train_labels))
print("Test score ", original_classifier.score(test_features, test_labels))
Train score 0.8
Test score 0.7
[18]:
original_classifier.save("c:\\test\\vqc_classifier.model")
[19]:
loaded_classifier = VQC.load("c:\\test\\vqc_classifier.model")
[20]:
loaded_classifier.warm_start = True
loaded_classifier.neural_network.sampler = sampler2
loaded_classifier.optimizer = COBYLA(maxiter=80)
[21]:
loaded_classifier.fit(train_features, train_labels)
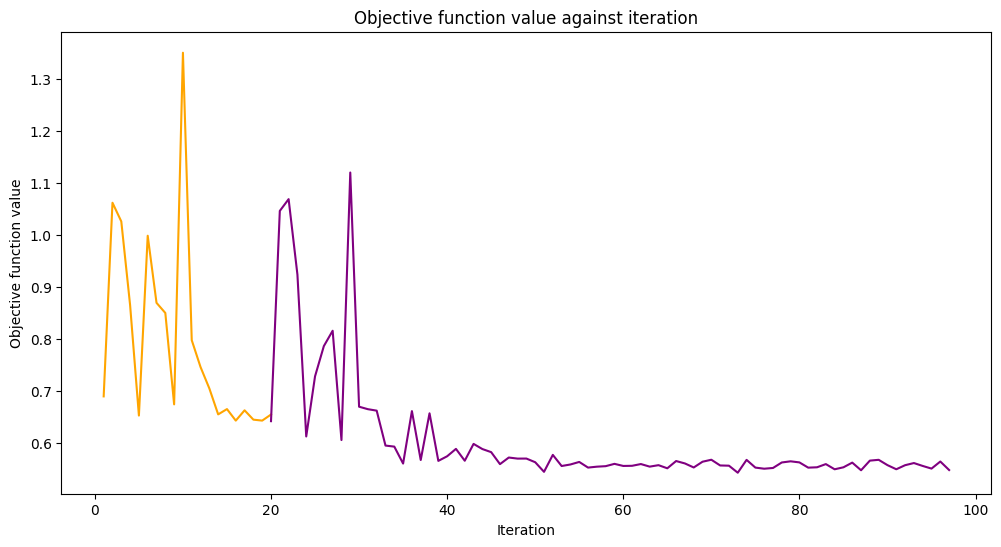
[21]:
<qiskit_machine_learning.algorithms.classifiers.vqc.VQC at 0x1ac0d0a2b10>
[22]:
print("Train score", loaded_classifier.score(train_features, train_labels))
print("Test score", loaded_classifier.score(test_features, test_labels))
Train score 0.8333333333333334
Test score 0.7
[23]:
train_predicts = loaded_classifier.predict(train_features)
test_predicts = loaded_classifier.predict(test_features)
[24]:
# return plot to default figsize
plt.rcParams["figure.figsize"] = (6, 4)
plot_dataset()
# plot misclassified data points
plt.scatter(
train_features[np.all(train_labels != train_predicts, axis=1), 0],
train_features[np.all(train_labels != train_predicts, axis=1), 1],
s=200,
facecolors="none",
edgecolors="r",
linewidths=2,
)
plt.scatter(
test_features[np.all(test_labels != test_predicts, axis=1), 0],
test_features[np.all(test_labels != test_predicts, axis=1), 1],
s=200,
facecolors="none",
edgecolors="r",
linewidths=2,
)
[24]:
<matplotlib.collections.PathCollection at 0x1ac0b9a4710>
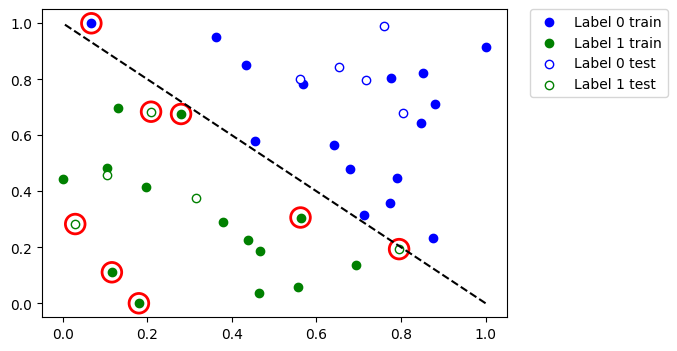
[ ]: