09 - Application Classes for Optimization Problems
[1]:
# This code is from the tutorial at:
# https://qiskit-community.github.io/qiskit-optimization/tutorials/09_application_classes.html
[2]:
from qiskit_optimization.algorithms import MinimumEigenOptimizer
from qiskit_algorithms.utils import algorithm_globals
from qiskit_algorithms import QAOA, NumPyMinimumEigensolver
from qiskit_algorithms.optimizers import COBYLA
# Switch to Quantum Rings's Sampler
#from qiskit.primitives import Sampler
from quantumrings.toolkit.qiskit import QrSamplerV1 as Sampler
Vertex cover problem
[3]:
from qiskit_optimization.applications.vertex_cover import VertexCover
import networkx as nx
seed = 123
algorithm_globals.random_seed = seed
graph = nx.random_regular_graph(d=3, n=6, seed=seed)
pos = nx.spring_layout(graph, seed=seed)
prob = VertexCover(graph)
prob.draw(pos=pos)
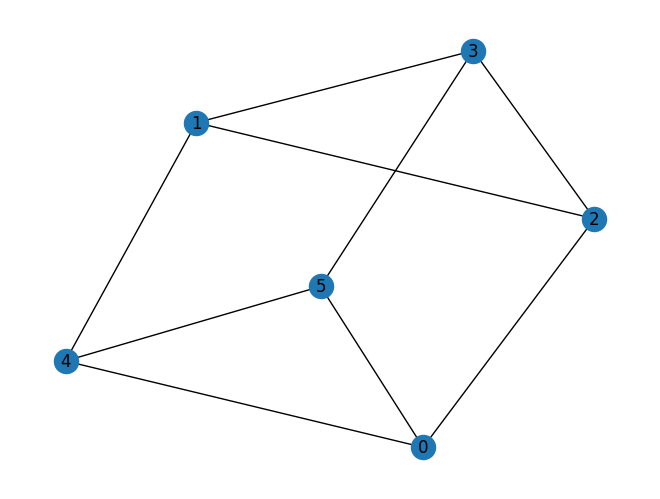
[4]:
qp = prob.to_quadratic_program()
print(qp.prettyprint())
Problem name: Vertex cover
Minimize
x_0 + x_1 + x_2 + x_3 + x_4 + x_5
Subject to
Linear constraints (9)
x_0 + x_4 >= 1 'c0'
x_0 + x_2 >= 1 'c1'
x_0 + x_5 >= 1 'c2'
x_1 + x_2 >= 1 'c3'
x_1 + x_4 >= 1 'c4'
x_1 + x_3 >= 1 'c5'
x_2 + x_3 >= 1 'c6'
x_3 + x_5 >= 1 'c7'
x_4 + x_5 >= 1 'c8'
Binary variables (6)
x_0 x_1 x_2 x_3 x_4 x_5
[5]:
# Numpy Eigensolver
meo = MinimumEigenOptimizer(min_eigen_solver=NumPyMinimumEigensolver())
result = meo.solve(qp)
print(result.prettyprint())
print("\nsolution:", prob.interpret(result))
prob.draw(result, pos=pos)
objective function value: 4.0
variable values: x_0=0.0, x_1=0.0, x_2=1.0, x_3=1.0, x_4=1.0, x_5=1.0
status: SUCCESS
solution: [2, 3, 4, 5]
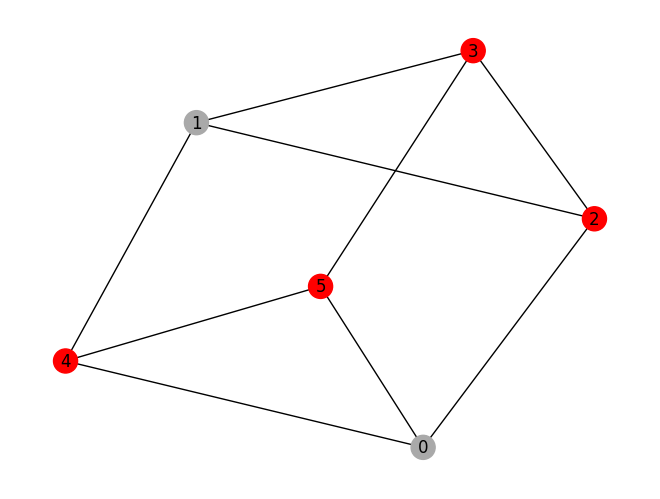
[6]:
# QAOA
meo = MinimumEigenOptimizer(min_eigen_solver=QAOA(reps=1, sampler=Sampler(), optimizer=COBYLA()))
result = meo.solve(qp)
print(result.prettyprint())
print("\nsolution:", prob.interpret(result))
print("\ntime:", result.min_eigen_solver_result.optimizer_time)
prob.draw(result, pos=pos)
objective function value: 4.0
variable values: x_0=1.0, x_1=1.0, x_2=0.0, x_3=1.0, x_4=1.0, x_5=0.0
status: SUCCESS
solution: [0, 1, 3, 4]
time: 1.6609249114990234
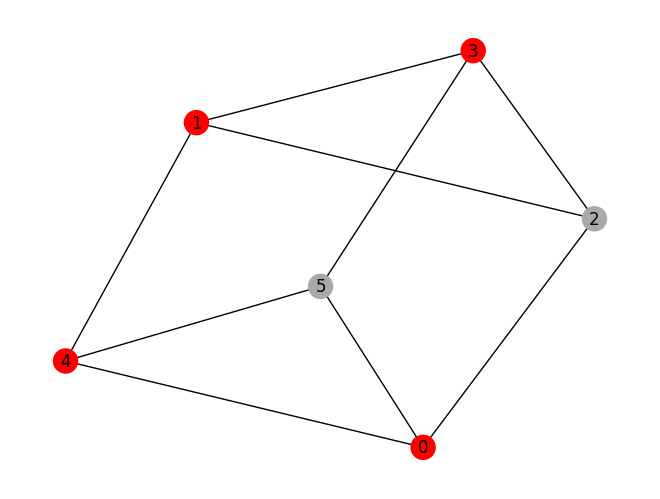
Knapsack problem
[7]:
from qiskit_optimization.applications import Knapsack
prob = Knapsack(values=[3, 4, 5, 6, 7], weights=[2, 3, 4, 5, 6], max_weight=10)
qp = prob.to_quadratic_program()
print(qp.prettyprint())
Problem name: Knapsack
Maximize
3*x_0 + 4*x_1 + 5*x_2 + 6*x_3 + 7*x_4
Subject to
Linear constraints (1)
2*x_0 + 3*x_1 + 4*x_2 + 5*x_3 + 6*x_4 <= 10 'c0'
Binary variables (5)
x_0 x_1 x_2 x_3 x_4
[8]:
# Numpy Eigensolver
meo = MinimumEigenOptimizer(min_eigen_solver=NumPyMinimumEigensolver())
result = meo.solve(qp)
print(result.prettyprint())
print("\nsolution:", prob.interpret(result))
objective function value: 13.0
variable values: x_0=1.0, x_1=1.0, x_2=0.0, x_3=1.0, x_4=0.0
status: SUCCESS
solution: [0, 1, 3]
[9]:
# QAOA
meo = MinimumEigenOptimizer(min_eigen_solver=QAOA(reps=1, sampler=Sampler(), optimizer=COBYLA()))
result = meo.solve(qp)
print(result.prettyprint())
print("\nsolution:", prob.interpret(result))
print("\ntime:", result.min_eigen_solver_result.optimizer_time)
objective function value: 13.0
variable values: x_0=1.0, x_1=1.0, x_2=0.0, x_3=1.0, x_4=0.0
status: SUCCESS
solution: [0, 1, 3]
time: 2.2351861000061035
How to check the Hamiltonian
[10]:
from qiskit_optimization.converters import QuadraticProgramToQubo
# the same knapsack problem instance as in the previous section
prob = Knapsack(values=[3, 4, 5, 6, 7], weights=[2, 3, 4, 5, 6], max_weight=10)
qp = prob.to_quadratic_program()
print(qp.prettyprint())
Problem name: Knapsack
Maximize
3*x_0 + 4*x_1 + 5*x_2 + 6*x_3 + 7*x_4
Subject to
Linear constraints (1)
2*x_0 + 3*x_1 + 4*x_2 + 5*x_3 + 6*x_4 <= 10 'c0'
Binary variables (5)
x_0 x_1 x_2 x_3 x_4
[11]:
# intermediate QUBO form of the optimization problem
conv = QuadraticProgramToQubo()
qubo = conv.convert(qp)
print(qubo.prettyprint())
Problem name: Knapsack
Minimize
26*c0@int_slack@0^2 + 104*c0@int_slack@0*c0@int_slack@1
+ 208*c0@int_slack@0*c0@int_slack@2 + 156*c0@int_slack@0*c0@int_slack@3
+ 104*c0@int_slack@1^2 + 416*c0@int_slack@1*c0@int_slack@2
+ 312*c0@int_slack@1*c0@int_slack@3 + 416*c0@int_slack@2^2
+ 624*c0@int_slack@2*c0@int_slack@3 + 234*c0@int_slack@3^2
+ 104*x_0*c0@int_slack@0 + 208*x_0*c0@int_slack@1 + 416*x_0*c0@int_slack@2
+ 312*x_0*c0@int_slack@3 + 104*x_0^2 + 312*x_0*x_1 + 416*x_0*x_2 + 520*x_0*x_3
+ 624*x_0*x_4 + 156*x_1*c0@int_slack@0 + 312*x_1*c0@int_slack@1
+ 624*x_1*c0@int_slack@2 + 468*x_1*c0@int_slack@3 + 234*x_1^2 + 624*x_1*x_2
+ 780*x_1*x_3 + 936*x_1*x_4 + 208*x_2*c0@int_slack@0 + 416*x_2*c0@int_slack@1
+ 832*x_2*c0@int_slack@2 + 624*x_2*c0@int_slack@3 + 416*x_2^2 + 1040*x_2*x_3
+ 1248*x_2*x_4 + 260*x_3*c0@int_slack@0 + 520*x_3*c0@int_slack@1
+ 1040*x_3*c0@int_slack@2 + 780*x_3*c0@int_slack@3 + 650*x_3^2 + 1560*x_3*x_4
+ 312*x_4*c0@int_slack@0 + 624*x_4*c0@int_slack@1 + 1248*x_4*c0@int_slack@2
+ 936*x_4*c0@int_slack@3 + 936*x_4^2 - 520*c0@int_slack@0
- 1040*c0@int_slack@1 - 2080*c0@int_slack@2 - 1560*c0@int_slack@3 - 1043*x_0
- 1564*x_1 - 2085*x_2 - 2606*x_3 - 3127*x_4 + 2600
Subject to
No constraints
Binary variables (9)
x_0 x_1 x_2 x_3 x_4 c0@int_slack@0 c0@int_slack@1 c0@int_slack@2
c0@int_slack@3
[12]:
# qubit Hamiltonian and offset
op, offset = qubo.to_ising()
print(f"num qubits: {op.num_qubits}, offset: {offset}\n")
print(op)
num qubits: 9, offset: 1417.5
SparsePauliOp(['IIIIIIIIZ', 'IIIIIIIZI', 'IIIIIIZII', 'IIIIIZIII', 'IIIIZIIII', 'IIIZIIIII', 'IIZIIIIII', 'IZIIIIIII', 'ZIIIIIIII', 'IIIIIIIZZ', 'IIIIIIZIZ', 'IIIIIZIIZ', 'IIIIZIIIZ', 'IIIZIIIIZ', 'IIZIIIIIZ', 'IZIIIIIIZ', 'ZIIIIIIIZ', 'IIIIIIZZI', 'IIIIIZIZI', 'IIIIZIIZI', 'IIIZIIIZI', 'IIZIIIIZI', 'IZIIIIIZI', 'ZIIIIIIZI', 'IIIIIZZII', 'IIIIZIZII', 'IIIZIIZII', 'IIZIIIZII', 'IZIIIIZII', 'ZIIIIIZII', 'IIIIZZIII', 'IIIZIZIII', 'IIZIIZIII', 'IZIIIZIII', 'ZIIIIZIII', 'IIIZZIIII', 'IIZIZIIII', 'IZIIZIIII', 'ZIIIZIIII', 'IIZZIIIII', 'IZIZIIIII', 'ZIIZIIIII', 'IZZIIIIII', 'ZIZIIIIII', 'ZZIIIIIII'],
coeffs=[-258.5+0.j, -388. +0.j, -517.5+0.j, -647. +0.j, -776.5+0.j, -130. +0.j,
-260. +0.j, -520. +0.j, -390. +0.j, 78. +0.j, 104. +0.j, 130. +0.j,
156. +0.j, 26. +0.j, 52. +0.j, 104. +0.j, 78. +0.j, 156. +0.j,
195. +0.j, 234. +0.j, 39. +0.j, 78. +0.j, 156. +0.j, 117. +0.j,
260. +0.j, 312. +0.j, 52. +0.j, 104. +0.j, 208. +0.j, 156. +0.j,
390. +0.j, 65. +0.j, 130. +0.j, 260. +0.j, 195. +0.j, 78. +0.j,
156. +0.j, 312. +0.j, 234. +0.j, 26. +0.j, 52. +0.j, 39. +0.j,
104. +0.j, 78. +0.j, 156. +0.j])
[ ]: